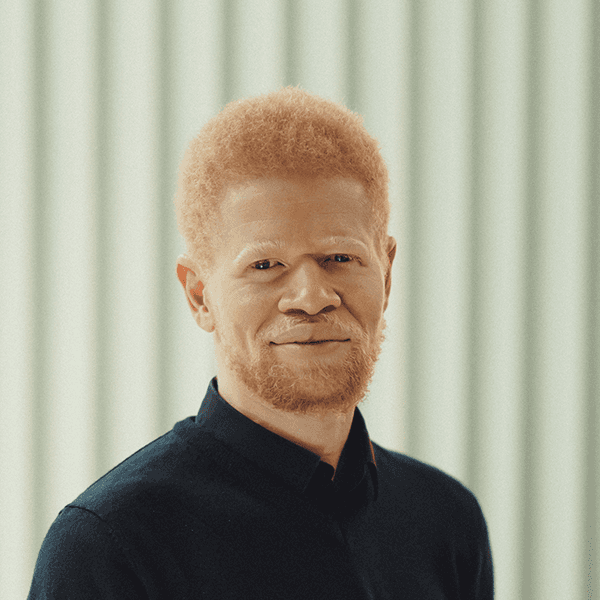
Joel Belafa
Oct 24, 2024
A few weeks ago, I had the opportunity to give a speech on AI's impact on business to a community of entrepreneurs and business leaders. This neutral exercise helped solidify an important idea: while loud promises about catchy business expectations often dominate the conversation, a beautiful silent revolution is occurring in many domains of expertise. The community that might benefit the most in the short term is likely researchers and scientists.
Future of Healthcare
Sustainable Healthcare Practices
Emerging Technologies
Artificial Intelligence
Health Big Data
Lifescience
Medical Devices
Dermocosmetics
Shaking the foundations of science
Science or not Science?
It's been a long time since research started to become data-driven. We gradually saw statistics and knowledge sharing rule the state of the art and decision-making in everything we could consider science.
While I'm reluctant to make strong statements about what is SCIENCE and what is not, Yann Lecun gathered the majority of AI researchers around the idea that research couldn't be "research" without peer review. The primary reason for peer review in science is to reduce the subjectivity of any approach or experiment conclusion.
Validating the consistency of research restitution means having the ability to:
Reproduce experiments or observations in different environments
Assess the plausibility of the main idea through pre-existing scientific knowledge
Once sufficient people and time are dedicated to challenging the hypothesis, if the large majority of evidence favors the idea, we could consider it "science.
AI and research: The infinite loop
Currently, there's a trend focusing on efforts to make AI reliable or at least assess its limitations. Given that Generative AI and transformers have unleashed a new wave of information processing and almost commoditized natural language processing (NLP), a fair share of our interaction with these models is to assess whether the sequence generation produced through statistical scoring reflects our knowledge.
This process is very close to how we build science. It's an infinite loop, as the moment the AI community gains confidence in a certain type of task, they move to a different and sometimes more difficult problem.
From saying to doing
Surprisingly, the escape route from this endless need for validation came from another use case with AI: the ability to use tools. The first argument behind the transformative impact of frontier models is the near-certainty that the vast majority of applications will be connected to one.
With the ability to use tools like browsing the internet, checking the weather, accessing internal databases, or running code, AI has gained the natural ability to double-check itself without human intervention. This is particularly evident in code execution, where AI can:
Write code
Run the code with a tool or in a given environment
Return any errors to the model, asking AI to fix them
Repeat the loop until the code runs without errors
What researchers needed most
Active assistance
Researchers, especially in life sciences, are generally careful and disciplined in their approach. Whether due to regulations or deontology, there's always a rule or best practice to follow. This disciplined approach has been the trigger for many good ideas we had when listing the potential benefits AI could bring to this space.
Reasoning & mutual understanding
There's obviously a challenge when tasks are so complex that they require multiple steps to achieve the ultimate goal. We've gradually managed to train AI to replicate our thinking process, using techniques like chain of thoughts for complex tasks. This expands the possibilities even further, allowing for the execution of very complex missions, including intermediate self-awareness, exit strategies, and parallel or adversarial research tasks.
Soft knowledge
The rules given to AI to follow and the means to assess each intermediate result must be human-readable and understandable. This is perhaps the most important benefit AI could bring to SCIENCE on a global scale. AI pipelines of tomorrow will be able to conduct experiments, isolate data points and root causes, and augment their own contextual knowledge with external sources.
The future of augmented research
The ultimate benefit of AI in research will be a constant fuel of knowledge, a constant challenge of any assumption, and a genuine explosion of productivity and progress. This could change the face of research and bring hope to big problems like drug discovery without jeopardizing general safety.
We need to allow AI to explore different paths, as long as we maintain the ability to convert any acquired knowledge into something we can verify from our observations. Think of this as replicating a space phenomenon on Earth with a special room – a simulation that would also benefit the model when it comes to transmitting concepts of the "real world" to solve problems with imperfect representations of the world.